Imagine increasing your sales in just one night. That’s exactly what we achieved with the Madison Mallards during their AI-powered game night. Here’s how we leveraged data to make it happen. In any business, data is a powerful tool. At Pandata Group, we're all about leveraging data to drive actionable insights. Recently, we were presented with a compelling challenge: to analyze and increase sales based on data from the Madison Mallards games, spanning 30 games this season. The data was used to craft targeted promotions aimed at boosting revenue.
How did we achieve it?
To tackle the challenge, we explored the datasets provided by the Madison Mallards Baseball Team. We started by analyzing the merchandise and food sales data, with the goal of identifying patterns that could formulate our promotional strategies.
We built a Machine Learning model which allowed us to uncover common trends and patterns, offering us valuable insights into sales behavior and preferences. Armed with this knowledge, we developed discount strategies tailored to each dataset, aiming to boost revenue.
The insights we gained helped the Mallards in implementing targeted promotions, ultimately leading to more effective marketing strategies and a significant boost in achieving their revenue objectives.
Let's dive deeper!
1. Data Collection and Exploration
We began by collecting and exploring two critical datasets:
- Merchandise sales summary data
- Food & Beverages sales summary data
The datasets included features such as Item Name, Item Variation, Category, Gross Sales, Items Refunded, Net Sales, and Refund Rate. Understanding these variables was essential for identifying potential opportunities for targeted promotions.
2. Data Cleaning
Cleaning the data is the first and most important step. The principle "Garbage In, Garbage Out" holds true - no matter how advanced the model inconsistent data is the bane of accurate analysis. Therefore, we meticulously cleaned the datasets to ensure consistency and accuracy. This process involved creating the final category, standardizing item names across multiple stalls, correcting unit discrepancies, and eliminating extraneous characters. By doing so, we ensured that our analysis was built on a solid foundation, free from the noise of data inconsistencies.
Raw data -> Final Category

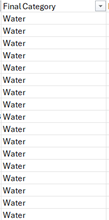
3. Feature engineering
To add more depth to our analysis, we engineered additional features that could provide nuanced insights into sales performance. For example, calculating the Refund Rate allowed us to gauge customer satisfaction and identify products that might benefit from promotional strategies. These derived features enriched our dataset, offering a clearer picture of sales dynamics.
4. Clustering analysis
With the data cleaned and enriched, we moved on to clustering analysis. By applying hierarchical clustering technique, we segmented products into distinct groups based on their sales characteristics. This analysis enabled us to uncover hidden patterns and relationships within the data, such as identifying which products had high sales but also high refund rates—an indicator of customer dissatisfaction or an opportunity for improved marketing.
Clusters formed with different Merch
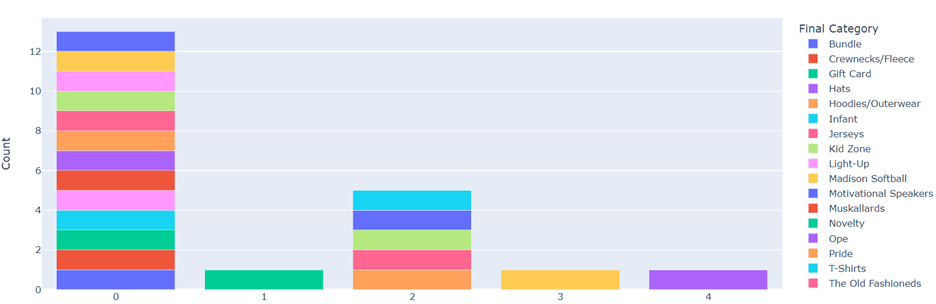
Clusters formed with different Food/Bev
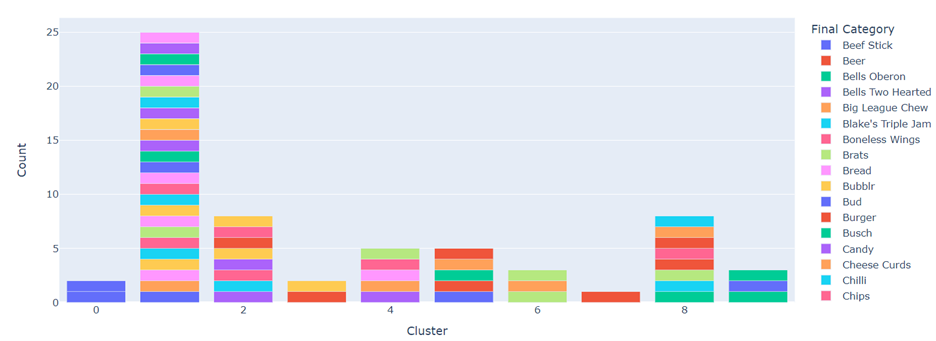
5. Post Clustering Analysis
Once clusters were made, it was time to understand the characteristics of each cluster and identify opportunities for targeted discounts - whether to apply discounts, run flash ads, or make no changes based on the cluster characteristics. We targeted discounts for products with high gross sales, high refund rates and low profitability.
Let’s look at the impact of promotions on sales! Alas! Our promotion recommendations are in. We provided a list of Products best suited for increasing revenue via promotions! Among those were donuts (Cinnamon Sugar donuts with Novelty hat, powdered donuts, large mini donuts), Pulled pork sandwich, Kona Big wave and Hats! Look at the percentage increase in units sold and net sales for this game
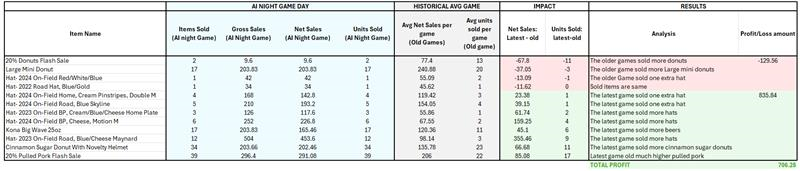
As we can see, on average 22 pulled pork sandwich was sold during a game. But during the Mallards AI night with promotions generated by our model they ended up selling 39 sandwiches, that’s a percent increase of 77%!
This achievement was the result of a collaborative effort across our team at Pandata Group, combining expertise in data science, business strategy, and marketing. The insights we provided helped the Mallards team implement targeted promotions, ultimately leading to more effective marketing strategies and a significant boost in achieving their revenue objectives.
Ready to harness the power of data for your business? Contact us at Pandata Group to explore how our data-driven strategies can drive your success.