Ever since December 2022, as Data and AI consultants, we have always been in calls with clients and business dinners, discussing how “Enterprise AI” can help businesses leverage the power of unstructured business data like text files, documents, images, emails, and customer reviews of companies or products. So, what is Gen AI, what does it mean for businesses, and is it all hype? Let’s explore these questions together.
What is GenAI in a nutshell?
“OpenAI”, “LLMs”, “AGI”, “GenAI”, “NVIDIA” - you may be living on a different planet (maybe Mars?) if you have not heard these terms in the past couple of years. To catch you up on the trend, remember these words: “RAG Systems” & “Agentic AI”.
“AGI” means artificial general intelligence, which is a capability of computers replicating human-level intelligence. Generative AI is a type of system that can create entirely new content, like text, images, or code, based on patterns it learns from large datasets, mimicking human-like creativity rather than just analyzing existing information.
In December 2022, when “OpenAI” released ChatGPT, a Large Language Model (“LLM”) text-based chatbot with capabilities for answering user questions and DALL-E for generating images based on user prompts, it left the world surprised. These models can process natural language in a more generalized way, like how humans process and respond to questions.
What does GenAI mean to businesses?
As we know, in the 21st century businesses and IT systems go hand in hand. Systematically formatting data into traditional databases, in turn powers transactional and analytic systems. As part of their operations, business also often collect files in unstructured formats like images, contracts and legal documents, feedback from customers over emails, and strategies for business execution.
GenAI, with a little added magic, can build systems that are smart and utilize these types of files to suitable business use cases like text generation, code generation, and creating strategies based on existing systems.
Widely Used Enterprise GenAI Architectures
We know that LLMs excel at summarizing text content, generating codes, and answering user-based questions. Traditional GenAI systems, or Large Language Models are systems trained on large amounts of data from open-source websites, textbooks, and knowledge systems that are publicly available. They do not have information on documents that are proprietary or internal.
Retrieval Augmented (RAG) based systems:

To leverage internal documents and process documents with the help of LLMs, Retrieval Augmented Generation is a search technique that ideally filters relevant information from the documents and passes it to LLMs to generalize the context to user-based prompts.
In the background, it chunks the data from multiple documents and embeds it into a vector database. The prompts from the user are also embedded into vector space to find the similar chunk from the existing documents. This will rank the chunks and pass it to the LLM as context for answering the questions in a generalized manner.
A use case of RAG includes chatbots that have access to HR documents where employees can ask questions such as: What is the dress code policy? Or, what are the current insurance plans that our company offers?
Agentic AI Systems:
Agentic AI systems are one step ahead in the GenAI space. These systems can create workflows with the help of AI agents. Say, if we want to extend the above use case of an employee interacting with the HR chatbot, which has information about his insurance policy details, the traditional AI systems will help him get his current plans and explain all existing plans in the company. Let’s take it one step further, if he wants to update his plan from “PPO” to “HSA,” the traditional LLMs could not handle these tasks. The agentic AI systems should be able to handle these tasks by creating workflows with multiple AI agents in between.
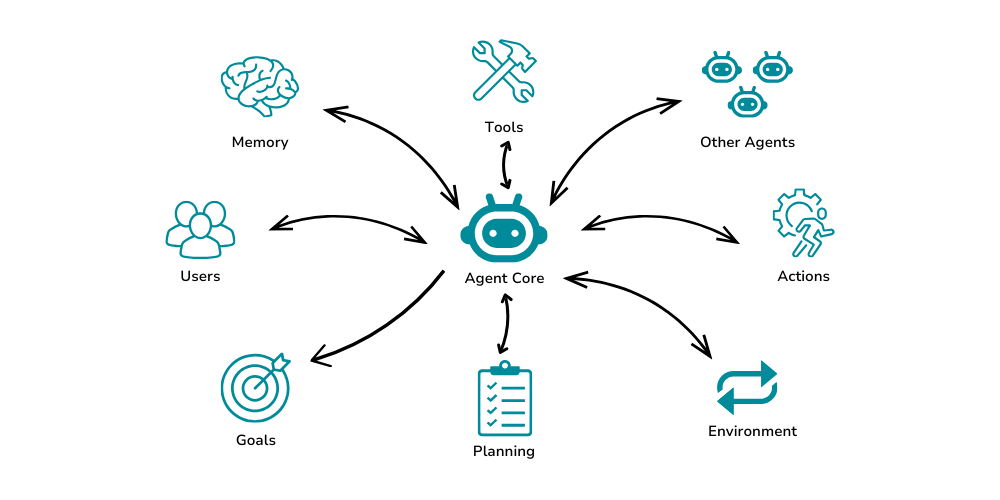
Another use case in the retail space can be if we have connected the AI agents to a database that has sales data; these agents can forecast sales and order necessary stock from suppliers to keep the business going.
What cloud platform should be used to build GenAI solutions?
Often, companies get confused about which cloud platforms or services should be used to build AI solutions. These solutions can be infrastructure, platform, or software as a service. The trade-off considerations to think about when making these decisions are the skills of IT employees and cost.
We can build solutions on the big 3 cloud providers; however, the major issues for clients are that this requires high level engineering skills and significant development time, and AI-based SaaS tools are highly priced.
So, is there any tool where we can prototype AI projects quickly and effectively and productionalize them on a reasonable budget?
Being a Snowflake partner, we got to meet the CEO of Snowflake, Mr. Sridhar Ramaswamy. His vision for Snowflake is as follows: “We wanted to democratize the AI research of Google’s, Meta’s, and Microsoft’s to businesses across the globe with added data governance and privacy.”
Snowflake Cortex AI offers GenAI products which can be used for building AI solutions with SQL and Python rapidly. We know that GenAI solutions are often complex and require a lot of skill in many technologies.
We have built multiple AI systems with Snowflake Cortex and the development experience has been hassle-free with minimal cloud costs. In particular, we have used Cortex Search based RAG systems to answer user questions on custom documents. Cortex Analyst is a self-service tool in which business users can ask questions in their natural language and receive direct answers from databases without writing SQL. Document AI, which is an object character recognition tool, is used to process invoices or documents and get target invoice values, dates, or other suitable values.

Speculation #1: Will GenAI replace human jobs?
Here’s the thing, businesses are leveraging and will continue to leverage GenAI. For example, Siemens Energy built a chatbot based on a retrieval-augmented generation (RAG) architecture on the Snowflake Data Cloud to quickly surface and summarize more than 700,000 pages of internal documents, helping accelerate research and development.
As a human, processing 700,000 pages of information is a tedious process; this use case shows how GenAI systems and humans working hand in hand can efficiently work together to build better processes.
To delve into more examples of GenAI use cases in industry, refer to our blog.
Speculation #2: Is GenAI expensive?
Companies should think about two important things when considering an investment in AI powered solutions.
Number one, return on investment (ROI). Let’s step back and think about a traditional analogy in the cloud world, “Memory is cheap; compute is not”. Let us also add accelerating computes (GPUs) to the picture. That means that this analogy is no longer true, but this one is: “Memory is cheap; compute may be cheap; but accelerated computing is not”.
We should be clear about ROI when making budget decisions for building AI systems. If the cost of building an AI system is more than a traditional solution, it’s likely not a proper use case at this point in time; as the cost for building and maintaining AI systems can get out of hand.
For example, a product manufacturing company building AI systems for customer agents that utilize product manuals, can help agents answer customer questions in a faster manner. This significantly decreases the time taken for customer agents to investigate the manuals and answer a customer.
Next, let’s consider utility. Utility refers to the value or benefit that a generative AI system delivers to users, businesses, or stakeholders, considering factors like cost benefits, efficiency gains, improved quality, and reduced risk. To find the utility, we need to clearly define the problem it aims to solve and identify the key metrics that represent the success of the project.
If we continue with the example of customer agents, answering customers creates greater customer satisfaction as we help them debug issues very quickly. In this case, the utility lies in enhancing customer satisfaction and enabling quicker responses from agents, which ultimately reduces time to value.
Speculation #3: Is GenAI just hype?
Companies should think about ROI and utility when building AI systems and consider adopting it now. Leading companies are taking steps to adapt and implement AI solutions, creating greater utility and furthering their competitive strategy. Adoption is key; don’t regret not getting on the train 5 years ago, 5 years from now.
Let’s Look Forward Together
Got questions on how to adopt AI and discuss ROI and utility of business needs in your organization? Let’s look forward together. We will help you board the AI train and discuss which solutions might work best for you, and when.