One of the most transformative advancements in recent years is Generative AI, a powerful technology that is revolutionizing how businesses operate, innovate, and create value. But what exactly is Generative AI, and how can it be leveraged to drive business success? In this article, we will demystify Generative AI, explore its potential applications, and highlight how it can be a value add for forward-thinking executives. Join us as we delve into the world of Gen AI and uncover the opportunities for your organization.
Generative AI refers to a subset of artificial intelligence techniques that involve creating new data or content based on patterns and examples from existing data. Unlike traditional AI systems that are typically designed for specific tasks like classification or prediction, generative AI models can generate novel outputs, such as images, text, music, and more.
As we know, the quest for developing intelligent machines existed from the early 1950’s. As we are in an era of producing data in huge volume, which is helping build models and systems which are intelligent by nature with help of Statistics, Machine Learning and Artificial Intelligence. As we speak, data is (more and more becoming) the fuel for building Machine Learning, Statistical and Artificial Intelligence Models.
Data, “The Fuel” of Gen AI systems:
Generative AI, at its core, relies heavily on data to function effectively. Data serves as the essential fuel that powers these advanced algorithms, enabling them to learn, generate, and innovate. Over the last several years the volume of data we generate daily has grown exponentially. Plus, thanks to complimenting technology advancements in cloud and cloud data storage we have a lot to work with.
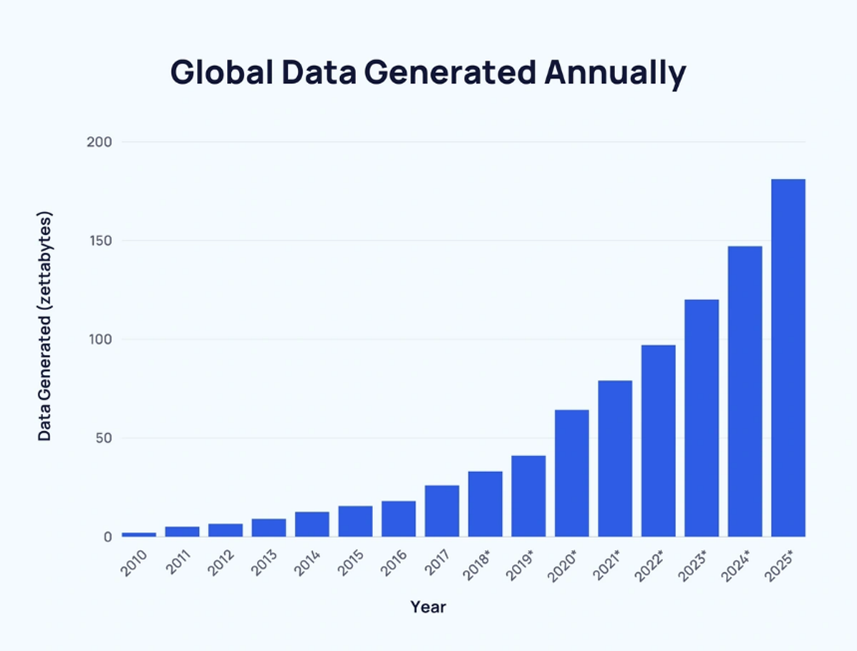
Generative AI models, such asGPT-4, are trained on vast amounts of data. This training data encompasses text, images, audio, and more, providing the foundational knowledge that the models use to generate new content. The more diverse and extensive the training data, the better the AI can understand and mimic complex patterns, leading to more accurate and realistic outputs.
During training, Generative AI models analyze the data to identify underlying patterns and structures. This learning process involves recognizing relationships, sequences, and nuances within the data. For instance, in natural language processing, the AI learns grammar, context, and semantics from text data, which it later uses to generate coherent and contextually appropriate text.
Once trained, Generative AI uses the learned patterns to generate new content. The quality and relevance of this content depend directly on the quality and diversity of the training data. High-quality data leads to more sophisticated and human-like outputs, while biased or incomplete data can result in inaccurate or skewed results.
Continuous access to new and varied data allows Generative AI models to refine their capabilities. By incorporating fresh data, the AI can adapt to emerging trends, languages, and contexts, improving its accuracy and performance over time. This iterative learning process ensures that the AI remains relevant and effective in adynamic environment.
In business applications, data specific to an industry, company, or project can be used to fine-tune Generative AI models. This customization allows the AI to generate outputs that are tailored to specific needs, enhancing its utility and value. For example, a Generative AI model trained on legal documents can produce more accurate legal texts, while one trained on marketing data can generate compelling promotional content.
How can we get started with Generative AI in our organization?
As the business collects a huge amount of data for running their day-to-day business operations, transactions, and manufacturing of products. The process of structuring data from different structures and converting them into data models is helping data analytics and AI initiative a new area of common ground, to utilize data for helping businesses in optimal business decision making.
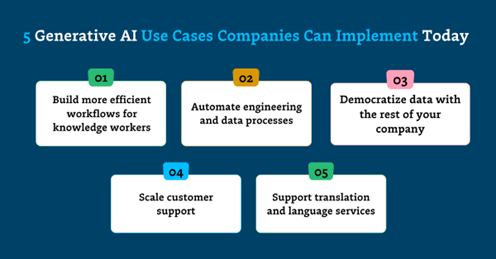
Generative AI has numerous applications across various sectors, revolutionizing traditional processes and offering innovative solutions. Here are some business use cases for Generative AI across different sectors:
1. Build more efficient workflows for knowledge workers:
- Businesses often have a lot of documentation that has been collected since their inception.
- The documentation can involve a collection of knowledge based on previous processes, operations, and employee findings.
- Moreover, the regulations of industries like legal, finance, and healthcare often change.
- With Gen AI, we can leverage the integration of business data and regulatory data, which can help and assist the employees of a business in answering their queries via prompts.
- This also helps them stay updated with regulations.
2. Automate engineering and data processes:
- There have been a lot of processes that can be automated with the help of generative AI.
- Code generation and integration in a pipeline can be automated with the advent of LLM-based code generation tools.
- This not only helps the development process happen quickly but also helps create mechanisms to automate the process of code review and quality improvement.
3. Democratizing data with the rest of your company:
- As we know, most traditional businesses collect and structure data in relational database models.
- Data modeling can get complex as it involves data and tables from multiple domains or subparts of businesses.
- Employees with a non-technical background need to rely on engineers to generate SQL or scripts, which can help them pull data from legacy systems. This process can be automated with Gen AI; we can generate scripts that can get data from database systems securely.
4. Scale Customer Support:
- Providing services and answering requests from customers is a tedious process as it involves real-time interaction and support.
- With Gen AI, we can create chatbots that can interact with users by providing answers to standard questions from the knowledge base with a human touch, as they can deliver responses like humans.
- We can also create a pipeline of language models that can answer customer support calls with Gen AI. Language and voice models can assist with a request and can also generate transcripts from the call.
5. Support translation and language services:
- Large Language Models from tech giants like OpenAI, Snowflake, and Google have mastered the art of content generation and image generation.
- Companies have been investing massively on creating unified translation models that can translate multiple languages into another language.
- This can also help businesses which operate in different regions or countries to translate communication via mail or real time translation in meetings.
Where to start and how to begin:
So, how do you take this idea of how Generative A.I. can be embedded into your business process for tangible outcomes? Pandata Group has established an A.I. practice with a team of passionate data enthusiasts, data scientists, and Gen AI engineers to prove out value creation from A.I.
Our methodology encompasses the entire lifecycle of any AI project. And that starts with a Value Creation exercise where we work with you to identify high-impact use cases that will have an immediate impact in a shorter period. This collaboration includes a rapid experimentation session to refine ideas and build proof-of-concepts (P.O.C.) for the most promising ones. These P.O.C.’s are all about efficiencies and focus on validating the core functionalities, avoiding unnecessary integrations or business rules.
For 10 years, we’ve been helping organizations modernize their data and analytics communities. The journey toward adopting and embedding Generative AI can impact customer interactions and streamline company operations. Connect with us to learn more about getting started with Gen AI.
Looking forward, our next discussion(s) will share more details on Snowflake’s evolution into an AI company, a focus on Retrieval-Augmented Generation (RAG), and a review of Large Language Models (LLMs).